
String rkUpperFilter = TableQuery.GenerateFilterCondition("RowKey", Quer圜omparisons.LessThan, "10") String rkLowerFilter = TableQuery.GenerateFilterCondition("RowKey", Quer圜omparisons.GreaterThanOrEqual, "5") String pkFilter = TableQuery.GenerateFilterCondition("PartitionKey", Quer圜omparisons.Equal, "samplePK") See the example "Sample – Query all entities with a PartitionKey=”SamplePK” and RowKey greater than or equal to “5”" at. There are two dedicated courses by Robert Cain on the Kusto Query Language on Pluralsight, which gives you deeper insight into KQL and that course is highly recommended for you as a data engineer as it details out the different kinds of commands and capabilities of KQL.A combined filter can then be combined with another filter, repeating as many times as necessary. These platforms also have saved queries that can be used to get an insight into how queries are formed and complex queries can be built.
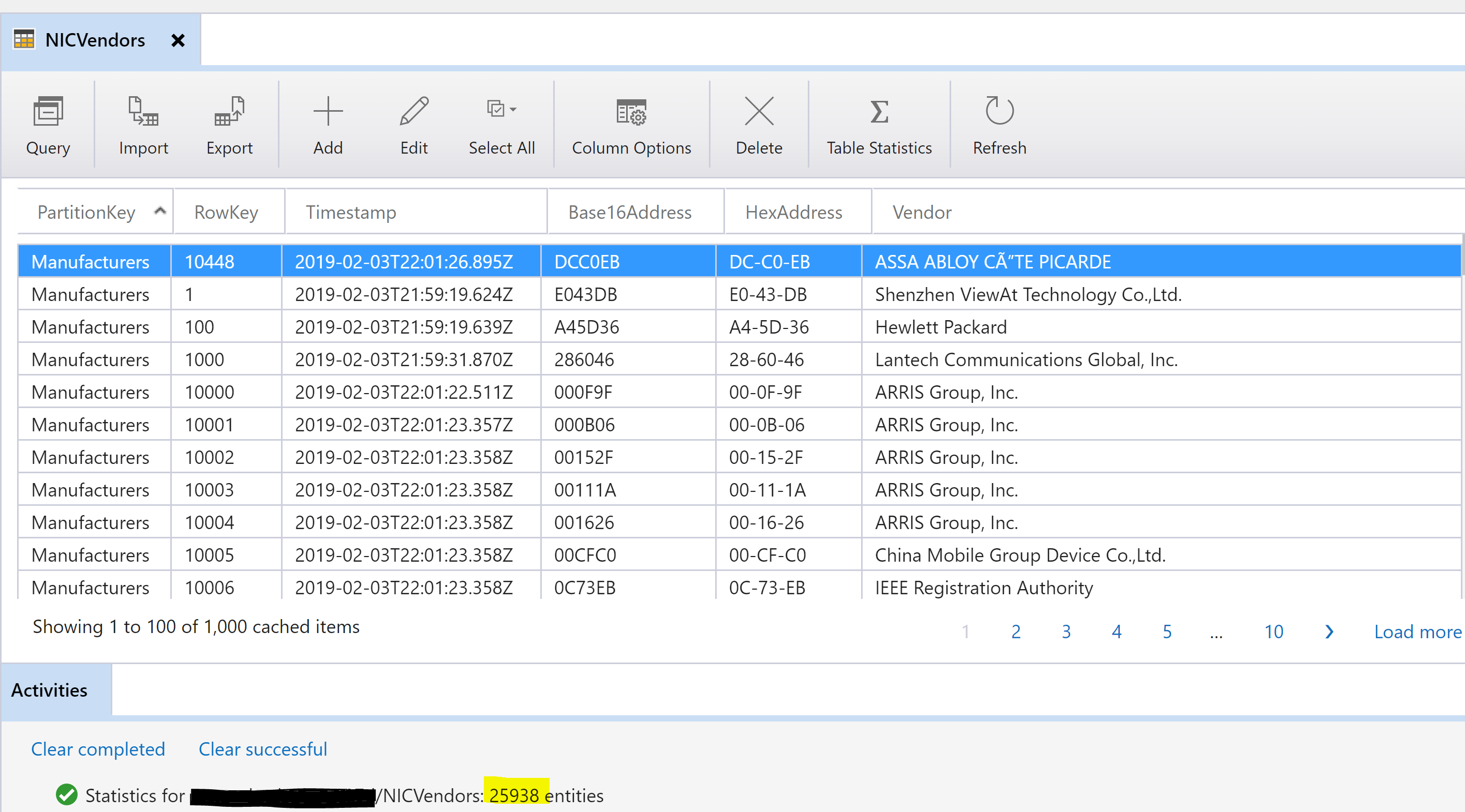

#Azure table query filter free#
There are certain demo platforms that are provided by Microsoft, which can be used free of cost for practice purposes. | summarize event_count = count() by State For this you can use Kusto to translate the SQL query to an equivalent KQL by prefixing it with ‘Explain’.

Also, the user can also get the KQL equivalent of the SQL command (in most cases), as KQL supports a subset of the SQL language. In order to know more about the data ingestion in Azure Data Explorer, visit Overview of Data Ingestion in Azure Data Explorer.Īnother interesting fact is that KQL knows to run the SQL commands as well. Data can also be ingested using Event Hub’s and Event Grid’s, and from the CSV file as well.
#Azure table query filter code#
It can be done using the custom code in any preferred language like Python.
#Azure table query filter windows#

KQL (Kusto Query Language) was developed with certain key principals in mind, like – easy to read and understand syntax, provide high-performance through scaling, and the one that can transition smoothly from simple to complex query. You can share the insights using Excel, or Power BI, or directly from the ADX. There are multiple different ways to share the visualized data. This can be done by exporting the data in the CSV format directly from ADX.įinally, after the data has been validated, the visualized data needs to be presented. This is where you would wish to share the data. We can use such queries to discover patterns, identify anomalies and outliers, create statistical modeling and more.Īt the end you should get your data validated by SMEs or stakeholders. This is technically called data ingestion.Īfter creating tables and ingesting data to them we can move forward and use Kusto Query Language (aka KQL) to explore the data. We already created the environment in the previous section, and now, we will extend our knowledge by first creating the tables using the Kusto explorer, and then import the data in the table from an external source.
